Deep learning (DL) has emerged as a critical Artificial Intelligence (AI) subfield, impacting diverse fields like natural language processing and computer vision. DL models rely on substantial hardware resources for efficient computation, especially when training extensive models on vast datasets. GPUs are essential for training these models due to their capacity for parallel processing. However, for researchers and organizations, the primary challenge is whether to purchase a dedicated GPU server or rent cloud-based GPU compute resources for running complex DL algorithms.
We'll assess the cost implications by comparing the financial and operational costs of dedicated GPU servers versus the costs associated with utilizing GPU-based cloud computing services.
Identifying your deep learning needs
Before delving into cost comparisons, you must fully grasp your project's specific needs. Firstly, consider the intricacies of the models; they range from being lightweight to highly complex. Similarly, gauge the volume of the dataset, which could be either modest or vast in size.
Additionally, the regularity of the training sessions can vary from sporadic to frequent iterations. These factors influence the type and capacity of GPUs needed and play a pivotal role in determining the overall project’ budget. Below, we compare dedicated on-premise vs. cloud computing costs across three key categories:
1. Initial investment and maintenance costs
Server:
- Hardware: Deep learning requires powerful hardware, particularly Graphics Processing Units (GPUs) for parallel processing. High-end GPU servers can cost a lot, with additional CPUs, memory, and storage costs. For example, the DGX A100 is suggested to cost around $200,000.
The costs of such systems reflect the inclusion of not only high-end GPUs but also substantial CPU, memory, and storage resources. For example, the DGX A100 features 1TB of system memory and 15TB of Gen4 NVMe internal storage.
- Infrastructure: Consider cooling systems and dedicated electrical circuits, adding thousands to the initial cost. High-performance GPUs generate significant heat and require effective cooling solutions to operate efficiently. Advanced cooling systems, whether they are air-cooled or liquid-cooled, are essential and can add considerably to the initial setup cost.
- Maintenance: Servers require regular maintenance including cleaning, hardware refresh, and software updates, which can be time-consuming and require IT expertise. These maintenance tasks are essential for ensuring optimal performance and longevity of the servers.
- Total cost of ownership (TCO): Calculate the cost of hardware, infrastructure, maintenance, electricity, cooling, and space over the server's lifespan for a complete picture.
Cloud computing:
- No upfront costs: The cloud's pay-as-you-go model eliminates the initial hardware and infrastructure burden, making it attractive for short-term projects or those with fluctuating resource requirements.
Thisl is especially attractive for short-term projects or those with fluctuating resource demands because it allows companies to scale resources up or down based on immediate needs without committing to long-term expenses.
- Variable costs: Users pay based on resource usage, including GPU type, memory size, and compute hours. While costs can start as low as cents per hour, extensive training can add up.
- Minimal maintenance: The cloud model also offloads some of the maintenance and management burden to the cloud service provider, including regular updates and system upkeep. This can further reduce the need for in-house IT expertise and allows organizations to focus more on their core business areas rather than on IT infrastructure management.
While these are great, there are other technical conderations to consider when choosing a cloud provider. Here are some of them:
- Virtualization: Cloud providers commonly employ server virtualization to maximize the efficiency of physical hardware. This technology allows multiple virtual machines (VMs) to operate on a single physical server, with each VM isolated and running its own operating system and applications.
However, this shared resource model can impact performance, especially when compared to using dedicated servers. Virtualized environments may experience variable performance due to the "noisy neighbor" effect, where other VMs on the same physical server consume disproportionate resources (CPU cycles, memory, disk I/O, network bandwidth), affecting the performance of adjacent VMs.
Understanding the specifics of a provider's virtualization technology and how they manage resource allocation is crucial. Providers typically offer different types of cloud service models, such as public, private, and hybrid clouds, each with varying levels of resource isolation, performance, and cost.
For instance, some cloud providers might use technologies like VMware or Hyper-V for virtualization, which include features designed to minimize the impact of resource contention. Others might offer dedicated instances or physically isolated hardware within a public cloud for performance-sensitive applications. Knowing these details can help users choose the right type of service based on their performance requirements and budget constraints.
- Networking: In cloud computing environments, particularly those used for data-intensive tasks such as training deep learning models, the speed and reliability of internet connections are critical factors that can significantly impact the effectiveness and efficiency of these processes.
Slow or unreliable internet can cause delays in data transmission, resulting in longer training times for models, especially when dealing with large datasets. This is because deep learning often require the transfer of vast amounts of data to and from the cloud. If the data cannot be uploaded, accessed, or downloaded swiftly, it can bottleneck the entire training process.
High-bandwidth internet connections are essential to mitigate these issues. For enterprises that rely heavily on cloud services for their data processing and machine learning tasks, investing in robust internet connectivity, or even dedicated lines, can be crucial to maximizing operational efficiency and model performance.
This reliance on strong internet connections highlights the need for careful planning regarding network infrastructure when deploying cloud-based AI and data analytics systems, especially for applications requiring real-time processing or large-scale data analysis.
2. Scalability and flexibility
Server:
- Scaling up: Scaling up hardware servers can be a cumbersome and expensive process. Adding additional hardware requires careful planning, integration, and configuration. Adding additional hardware is not as straightforward as it may seem—it requires significant planning, integration, and configuration.
- Planning: Expanding server capacity often involves evaluating the current and future needs to ensure that the new hardware will adequately meet projected demands. This planning phase can include assessments of power requirements, space, cooling capacity, and budget allocations.
- Integration: Adding new hardware to existing systems must be done with consideration for compatibility with existing components. This can involve firmware updates, ensuring that new hardware is compatible with existing operating systems and applications, and sometimes even changes to network configurations.
- Configuration: Once new hardware is physically installed, it must be properly configured. This includes setting up system parameters, network settings, and installing or updating software. Configuration also often requires extensive testing to ensure that the new hardware integrates smoothly with the existing system without causing disruptions.
- Scaling down: Scaling down unused hardware becomes a financial burden. Downsizing a server often entails selling components at a loss. Downsizing server hardware isn't merely a logistical challenge—it also involves financial considerations that can impact an organization's technology budget.
- Depreciation: Hardware components, such as servers, generally depreciate over time. The rapid pace of technological advancements can quickly render older models obsolete or less desirable, reducing their market value significantly.
- Resale market: The market for used IT equipment can be volatile. Factors such as supply and demand, the release of newer technology, and the condition of the equipment all play critical roles in determining resale value. Typically, companies can expect to sell off their used hardware at a substantial loss compared to their original purchase price.
- Logistics and costs: The process of decommissioning, preparing, and selling used hardware also incurs costs. This includes the labor involved in safely removing and preparing the equipment for sale, as well as potential costs associated with storage and transportation.
- Environmental considerations: Companies must also consider the environmental impact of disposing of old hardware. Proper disposal might require recycling or refurbishing, which can further add to the costs, though it is crucial for minimizing environmental impact.
- Limited resource pool: Llimited hardware options on a server can restrict the types of deep learning projects it can handle effectively. Expanding capabilities necessitates a complete hardware overhaul.
Cloud computing:
- Dynamic scaling: This feature of cloud computing allows users to adjust computing resources such as GPUs, memory, and storage based on the current needs of their projects. Scaling can typically be managed through a straightforward user interface on the cloud platform. This capability ensures that resources are not wasted, as users can scale down during periods of low demand and scale up during peaks, thus optimizing costs and efficiency.
- Elasticity: Cloud computing provides the ability to access a vast pool of resources, which is essential for handling larger or more complex computational tasks on demand. This is particularly beneficial for research and development projects that may have evolving requirements. Elasticity ensures that projects can be scaled appropriately without the need for upfront investments in physical infrastructure.
- Flexibility in hardware: The cloud allows users to select specific types of hardware that best fit their project's requirements. For instance, certain deep learning tasks might benefit more from GPUs with high-bandwidth memory, such as those equipped with NVIDIA's Tensor Cores, while others might require more raw processing power or specific types of CPUs. This flexibility helps optimize performance and cost, as users can tailor the hardware to the application’s needs without being locked into one configuration.
3. Performance and efficiency
Server:
- Hardware choice: Organizations have complete control over hardware selection is a significant advantage for on-premise servers. They can choose specific GPUs, balance memory bandwidth, and optimize storage performance to maximize efficiency for particular tasks. This customization can lead to better-tailored systems that are highly efficient for specific deep learning operations.
- Potential Obsolescence: Rapid advancements in GPU technology can render a server outdated. The pace of innovation in GPU technology is swift, with major manufacturers like NVIDIA and AMD frequently releasing new models that offer substantial improvements in processing power, energy efficiency, and capabilities (like enhanced AI-driven functionalities). Each new generation of GPUs often brings considerable performance enhancements, which can make previous models less efficient or inadequate for cutting-edge applications.
Cloud computing:
- Cutting-edge hardware: Cloud providers often maintain the latest hardware configurations, frequently updating their GPU offerings. This setup ensures that users have access to the most advanced hardware without the need for continuous personal investment in new technology. This can be particularly beneficial for deploying state-of-the-art deep learning models that require the latest computational capabilities.
- Optimized software stacks: Many cloud providers optimize their environments with the latest versions of deep learning frameworks and libraries, such as TensorFlow, PyTorch, and cuDNN. This optimization is designed to maximize the performance of the available hardware, offering enhanced efficiency and potentially reducing the time and effort required for configuration and maintenance.
- Shared resources: While cloud computing offers scalability and access to top-tier hardware, performance can fluctuate due to the shared nature of resources. Understanding the specifics of a cloud provider's resource allocation policies (dedicated vs. shared instances) is crucial. Additionally, cost-saving options like spot instances might offer financial benefits, but they come with the risk of interruptions, which could impact long-running deep learning tasks.
4. Security and data privacy
Server:
- Greater control: Users completely control physical security measures and data access protocols. This can be crucial for highly sensitive projects or those with strict regulatory compliance requirements.
- Management burden: Maintaining robust security measures requires ongoing effort, including software patching, vulnerability management, and user access control.
Cloud computing:
- Shared responsibility model: Security is a shared responsibility between the provider and the user. Providers are responsible for securing their infrastructure, while users are responsible for securing their data and configurations within the cloud environment.
- Compliance certifications: Many cloud providers offer compliance certifications relevant to specific industries (e.g., HIPAA for healthcare). These certifications provide peace of mind when handling sensitive data.
- Potential vendor lock-in: Migrating data and workloads between cloud providers can be complex, leading to concerns for vendor lock-in.
The choice between a server and cloud computing for deep learning infrastructure hinges on several factors. Consider your project's specific needs regarding budget, scalability, performance requirements, and security concerns.
Cloud computing might be ideal for budget-conscious projects with limited upfront costs and fluctuating resource requirements.
A server might be preferable for projects requiring complete control over hardware and security.
For research projects with evolving demands, the scalability and elasticity of the cloud offer significant advantages.
Related: 5 Best and Most Cost-Effective Deep Learning GPU Systems
How does CUDO Compute support deep learning projects?
CUDO Compute is designed to cater to the demanding nuances of deep learning in both efficiency and cost-effectiveness. Let's delve deeper into the platform’s distinguishing attributes:
Optimized GPU utilization: Beyond merely offering access to scarce GPU resources, CUDO Compute lets users take advantage of previously untapped computing resources spread across an extensive global network. This means that when you rent GPU hours, you rely on hardware located closer to you, eliminating latency and enhancing network responsiveness.
Flexible Pricing: Recognising the diverse ML needs of our users, we offer a competitive and versatile pricing strategy. Whether you're an individual researcher dabbling in occasional ML projects or a sprawling enterprise with consistently high computational demands, the pricing models are tailored to ensure you're charged based on your consumption.
Support for leading deep learning frameworks: CUDO Compute stays abreast of deep learning trends and offers compatibility with popular frameworks like TensorFlow. This ensures that transitioning or integrating into your established workflows is as smooth as possible.
Security: In an era where data breaches are becoming increasingly common, we place paramount importance on data integrity and security. The platform implements stringent security protocols, assuring users that their data remains invulnerable.
Ultimately, choosing between GPU-based cloud computing and purchasing a dedicated server for deep learning depends on the specific demands of your project. Purchasing dedicated GPUs may incur high costs, but it could prove cost-effective in the long run as your project duration increases.
About CUDO Compute
CUDO Compute is a fairer cloud computing platform for everyone. It provides access to distributed resources by leveraging underutilized computing globally on idle data center hardware. It allows users to deploy virtual machines on the world’s first democratized cloud platform, finding the optimal resources in the ideal location at the best price.
CUDO Compute aims to democratize the public cloud by delivering a more sustainable economic, environmental, and societal model for computing and empowering businesses and individuals to monetize unused resources.
Our platform allows organizations and developers to deploy, run, and scale based on demands without the constraints of centralized cloud environments. As a result, we realize significant availability, proximity, and cost benefits for customers by simplifying their access to a broader pool of high-powered computing and distributed resources at the edge.
Continue reading
5 mistakes to avoid in AI infrastructure projects: from inefficient training to poor planning
16 min read
Real-world benchmarks demonstrating performance variances across different GPU cloud infrastructures
11 min read
Choosing a GPU cloud provider in 2025: A proven evaluation checklist
25 min read
How to select the right GPU for your AI workload
18 min read
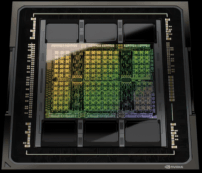
High-performance cloud GPUs